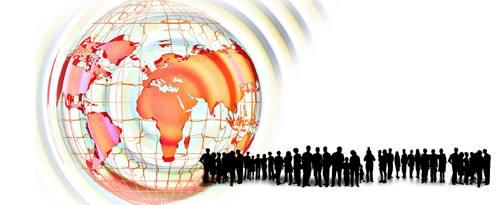

sportytrader jogos de hoje
O clube oferece aos seus usuários uma ampla variedade de esportes para apostar, desde os mais tradicionais. como futebol e 😊 basquete ou tênis; até outros menos conhecidos - com jogos eletrônicos da política”. Isso permite que o usuário escolham as 😊 mercados em sportytrader jogos de hoje onde estão muito interessadose aumenteram suas chances De ganhar
Além disso, o Bet sport 360 inclub oferece a 😊 seus usuários uma plataforma segura e confiável para realizar suas aposta. O clube é licenciadoe regulamentado por autoridades respeitadas do 😊 setor;o que garante de as jogadaS sejam justam E transparentees!
Outra vantagem do Bet sport 360 oclub é sportytrader jogos de hoje plataforma fácil 😊 de usar e intuitiva. Independentemente pelo nível da experiência dos usuário, É facilmente navegar no site ou fazer apostaas! O 😊 clube também oferece aos seus usuários recursos educacionais úteis - como dicas com conselhos para probabilidade a),para ajudá-losa tomar decisões 😊 informadas”.
Em resumo, o Bet sport 360 inclub é uma excelente opção para quem deseja entrar no mundo dos jogos de 😊 azar esportivo. Com sportytrader jogos de hoje ampla variedadede esportes e mercados em sportytrader jogos de hoje Uma plataforma segura E confiável com recursos educacionais úteis; 😊 este clube oferece tudo O que um apostador precisa pra ter sucesso!
tar. O pontapé de saída está programado para as 18:00 hora local no domingo 18
São 15:00 GMT- horário do 💷 Canadá, 7:00 horário de Pacífico na costa oeste dos EUA e
00 Horário do leste na Costa Leste dos Estados Unidos. 💷 Como assistir Argentina vs
2024 FIFA World Cup final ao vivo... olympics : notícias do
05:00 EST UK 10:00 GMT Que